Lets explore Data Scientist vs. Data Engineer. In the dynamic global of data-driven decision-making, the jobs of data scientists and data engineers play a key role in extracting precious insights from overall information. Both professions are essential to fulfilling any data-centric company, but they’re distinct in their objectives, skill sets, and duties. In this comprehensive article, we discover the differences between data scientists and data engineers, shedding light on their particular contributions to the world of data science.
What is a Data Scientist?
Data scientists are the masterminds behind uncovering meaningful styles and traits from complex datasets. They own a concentrated historical past in information, mathematics, and programming, enabling them to control and analyze records to extract precious insights. The primary responsibility of a data scientist is to convert raw records into actionable statistics, which can be used to make knowledgeable business selections and power growths.
Core Responsibilities of a Data Scientist
Data Analysis and Interpretation: Data scientists possess advanced analytical skills, using diverse statistical strategies and device-mastering algorithms to discover, interpret, and derive patterns from datasets.
- Predictive Modeling: Utilizing machine-getting-to-know algorithms, statistics scientists build predictive models to forecast future traits, behavior, and effects.
- Data Visualization: Sharing complex insights correctly is critical, and data scientists excel at growing visually appealing and understandable information visualizations, assisting in decision-making processes.
- Business Insights: Collaborating with stakeholders, data scientists identify business challenges and provide data-driven solutions to improve operational efficiency and optimize processes.
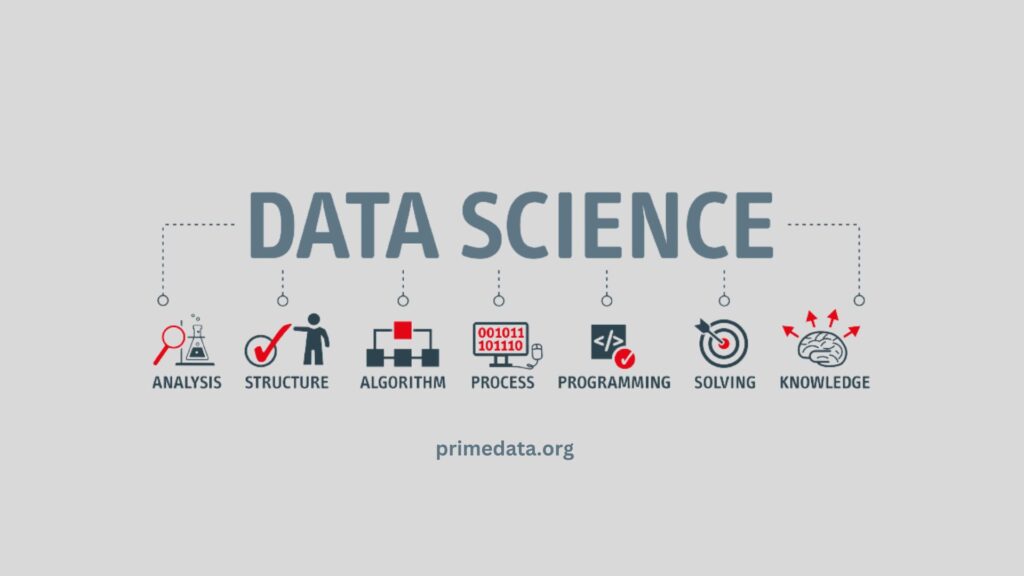
Skills Required for a Data Scientist
- Strong proficiency in programming languages such as Python, R, or SQL.
- Expertise in machine learning algorithms and statistical analysis.
- Familiarity with data visualization tools like Tableau, Power BI, or Matplotlib.
- Good communication skills to convey complex findings to non-technical audiences.
What is a Data Engineer?
While data scientists focus on extracting insights from data, data engineers lay the groundwork by designing and maintaining the data infrastructure that enables efficient data storage, recovery, and processing. Data engineers are skilled in building and optimizing data pipelines, ensuring that data is readily available and accessible for analysis by data scientists and other stakeholders.
Read More: The Ultimate Guide: 15 Data Science Tools You Can’t Ignore
Core Responsibilities of a Data Engineer
- Data Infrastructure: Data engineers are accountable for designing, building, and maintaining facts pipelines, data warehouses, and databases to handle huge amounts of structured and unstructured statistics.
- Data Integration: They integrate various data resources, ensuring seamless statistics go with the flow throughout the agency and allowing data scientists to get entry to relevant records for analysis.
- Performance Optimization: Data engineers optimize records systems for velocity, performance, and reliability, considering brief and accurate statistics retrieval.
- Data Quality and Security: Data integrity and security are vital, and data engineers enforce measures to protect sensitive statistics and keep records best.
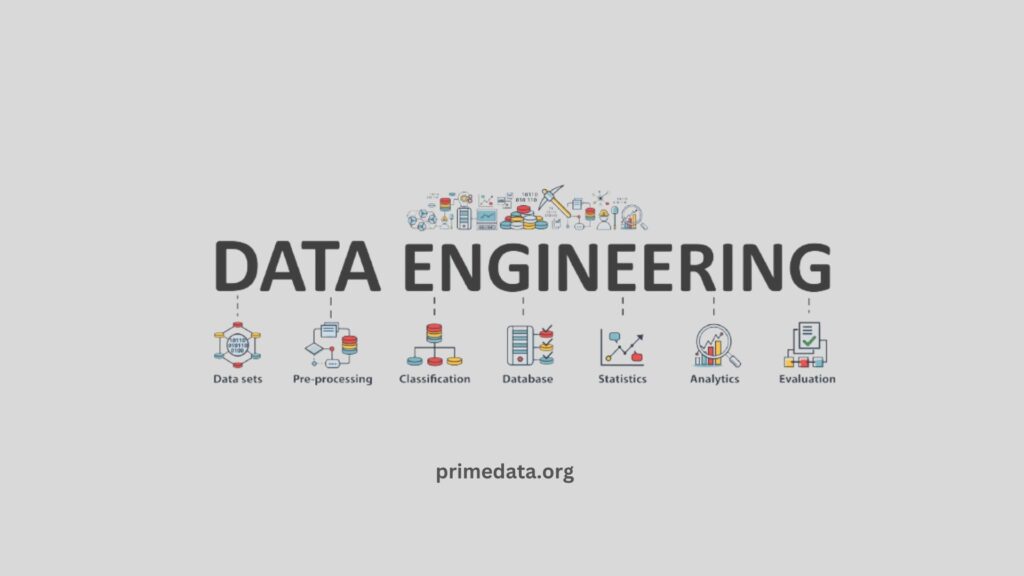
Skills Required for a Data Engineer
- Proficiency in programming languages like Python, Java, or Scala.
- Knowledge of distributed computing structures like Apache, Hadoop, or Spark.
- Expertise in statistics modeling and database management structures like MySQL, MongoDB, or Cassandra.
- Familiarity with cloud systems which includes AWS, Azure, or Google Cloud.
Data Scientist vs. Data Engineer
The difference between scientists and engineers ought to now not be mistaken for a divide; as an alternative, their roles complement every difference in achieving data-driven achievement. Collaboration among these roles is important for powerful data-pushed decision-making.
Data scientists depend on data engineers to provide them with easy, well-dependent data that are easy on the hand and available for analysis. Conversely, data engineers depend on facts scientists to communicate the enterprise necessities and insights they need to implement the best statistics infrastructure.
Can a Data Scientist Become a Data Engineer?
Absolutely! A Data Scientist can transition into a Data Engineer role with the right skill development and training. While Data Scientists and Data Engineers have distinct responsibilities, their skill sets often overlap, making the transition smoother.
Here are the key steps a Scientist can take to become a Data Engineer:
- Gain Programming Skills: Engineers typically work with programming languages like Python, Java, Scala, or SQL. Data Scientists already familiar with Python or R have a head start. To become a Data Engineer, it’s crucial to deepen your programming knowledge and study extra approximately building data pipelines and statistics integration.
- Learn Database Management: Engineers work substantially with database management systems like MySQL, PostgreSQL, MongoDB, and others. Understanding layout, preserving, and optimizing databases for a hit transition.
- Explore Big Data Technologies: Familiarize yourself with large facts technologies like Apache Hadoop, Apache Spark, and dispensed computing frameworks. Data Engineers regularly cope with massive volumes of records, and scalability in these technologies is valuable.
- Controller Data Modeling and ETL: Extract, Transform, and Load (ETL) processes are essential for data engineering. Understanding data modeling techniques and ETL best practices will be instrumental in the transition.
- Gain Cloud Platform Experience: Many organizations are moving their data infrastructure to cloud platforms like AWS, Azure, or Google Cloud. Acquiring experience with these cloud services is highly beneficial for Data Engineers.
- Work on Personal Projects: Engaging in personal data engineering projects can help solidify your skills and build a portfolio to showcase to potential employers.
- Take Online Courses or Training: Many online platforms offer data engineering courses and certifications. Enrolling in such courses can provide structured learning and industry-relevant skills.
- Seek Mentorship and Networking: Connecting with experienced Data Engineers, seeking mentorship, and participating in relevant industry events or meetups can provide valuable insights and guidance during your transition.
Remembering that transitioning from Data Scientist to Data Engineer might take time and effort. However, the analytical and problem-solving skills acquired as a Data Scientist can be advantageous in Data Engineering. A Data Scientist can pivot to a rewarding career as a Data Engineer by continually learning and developing new skills.
Read More: What Is Structured Data? A Comprehensive Guide
Conclusion
In conclusion, data scientists and data engineers are both indispensable players in the world of data science. While data scientists know about studying and interpreting facts to derive meaningful insights, data engineers construct and keep the infrastructure necessary for efficient information processing. Their collaboration is essential for corporations to harness the energy of records and make knowledgeable, statistics-driven decisions.
By expertise in the key differences between data scientists and engineers, companies can better optimize their facts groups and leverage their unique strengths to propel their fulfillment inside the generation of information analytics.
Remember, a well-established and collaborative statistics crew is the foundation of any successful facts-pushed corporation. So, spend money on building a cohesive crew that mixes the know-how of facts, scientists, and engineers to free up the proper capability of your records property.
Frequently Asked Questions (FAQs)
What does a Data Scientist do?
Data Scientists analyze and interpret facts using statistical and machine-mastering strategies to derive treasured insights and make informed enterprise selections.
What are the primary responsibilities of a Data Engineer?
Data Engineers layout and maintain facts infrastructure, information pipelines, and databases to ensure green facts garage, retrieval, and processing.
Can a Data Scientist become a Data Engineer and vice versa?
Yes, both roles share overlapping skills, allowing professionals to transition between Data Scientist and Data Engineer positions with the right training.
What programming languages are essential for Data Scientists and Data Engineers?
Data Scientists often use Python or R, while Data Engineers typically work with Python, Java, or Scala.
How do Data Scientists and Data Engineers collaborate?
Data Scientists rely on Data Engineers to provide them with clean, accessible data for analysis, and Data Engineers depend on Data Scientists’ insights to implement suitable data infrastructure. Collaboration ensures effective data-driven decision-making.